-
Financial administration
An accurate financial administration provides you with the information you need to take the right decisions. The big advantage of a digital financial administration is that it provides insight into your most important financial processes at any time, whether this is the invoices, salary payments or bank changes.
-
Financial insight
You want to take the right decisions, based on trustworthy and clear management information. You want to have access to all your financial data, 24/7, in order to determine your position and be able to adjust where necessary.
-
Global compliance partnering
Outsourced compliance services comprises the total financial compliance of your business, in accounting, financial reporting, payroll, legal and various tax reporting obligations. We can make sure you don’t have to worry.
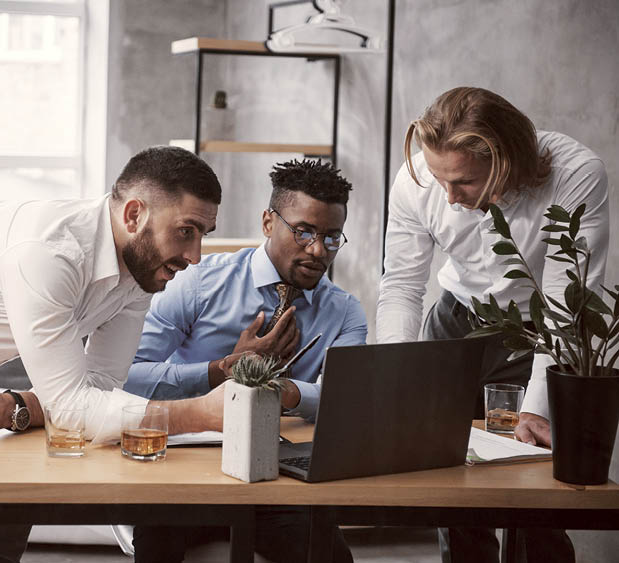
-
Business risk services
Minimize risk, maximize predictability, and execution Good insights help you look further ahead and adapt faster. Whether you require outsourced or co-procured internal audit services and expertise to address a specific technology, cyber or regulatory challenge, we provide a turnkey and reliable solution.
-
Corporate finance
Finding a suitable match at the most optimum terms. That, in a nutshell, aptly describes the objective of mergers and acquisitions. To most businesses mergers or acquisitions are not standard daily practice. It is, however, for the professionals at Grant Thornton! Seeking their services will add value instantly.
-
Cyber risk services
What should I be doing first if my data has been kidnapped? Have I taken the right precautions for protecting my data or am I putting too much effort into just one of the risks? And how do I quickly detect intruders on my network? Good questions! We help you to answer these questions.
-
Impact House
Building sustainability and social impact. That sounds good. But how do you go about it in the complex world of stakeholders, regulations and frameworks and changing demands from clients and society? How do you deal with important issues such as climate change and biodiversity loss?
-
Transaction services
What will the net proceeds be after the sale? How do I optimise the selling price of my business or the price of one of my business activities? How do I capitalise on synergies following an acquisition? Am I not offering too much? These are all good questions when you’re buying or selling a business. It’s a transaction that concerns significant amounts, impacts your future, and therefore must be executed properly. We provide a solid foundation for your decisions.
-
Valuation, investigation & dispute services
Do you require a fact finding investigation to help assess irregularities? Is it necessary to ascertain facts for litigation purposes?
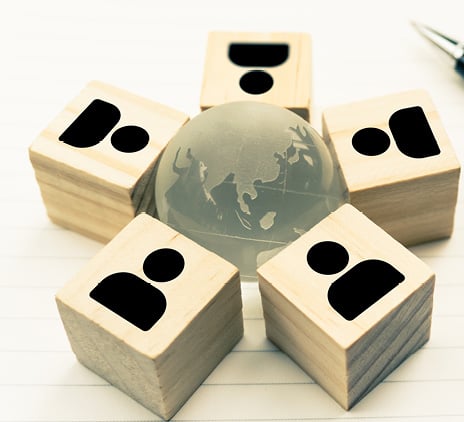
-
Auditing of annual accounts
You are answerable to others, such as shareholders and other stakeholders, with regard to your financial affairs. Financial information must therefore be reliable. What is more, you want to know how far you are progressing towards achieving your goals and what risks may apply.
-
IFRS services
Financial reporting in accordance with IFRS is a complex matter. Nowadays, an increasing number of international companies are becoming aware of the rules. But how do you apply them in practice?
-
ISAE & SOC Reporting
Our ISAE & SOC Reporting services provide independent and objective reports on the design, implementation and operational effectiveness of controls at service organizations.
-
Pre-audit services
Pre-audit services is all about making the company’s entire financial administration ready for checking before the external accountant begins his/her audit of the annual accounts.
-
SOx law implementation
The SOx legislation dictates that management is structurally accountable for reporting on the internal control relevant to the financial statements.
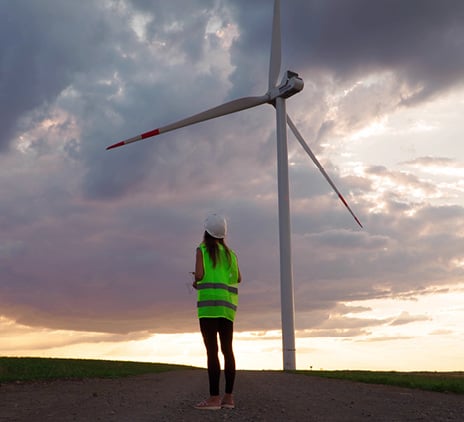
-
International corporate tax
The Netherlands’ tax regime is highly dynamic. Rules and the administrative courts raise new challenges in fiscal considerations on a nearly daily basis, both nationally and internationally.
-
VAT advice
VAT is an exceptionally thorny issue, especially in major national and international activities. Filing cross-border returns, registering or making payments requires specialised knowledge. It is crucial to keep that knowledge up-to-date in order to respond to the dynamics of national and international legislation and regulation.
-
Customs
Importing/exporting goods to or from the European Union involves navigating complicated customs formalities. Failure to comply with these requirements usually results in delays. In addition, an excessively high rate of taxation or customs valuation for imports can cost you money.
-
HR services
Do your employees determine the success and growth of your organisation? And are you in need of specialists which you can ask your Human Resources (HR) related questions? Human Resources (HR) related questions? Our HR specialists will assist you in the areas of personnel and payroll administration, labour law and taxation relating to your personnel. We provide you with high-quality personnel and payroll administration, good HR guidance and the right (international) advice as standard. All this, of course, with a focus on the human dimension.
-
Innovation & grants
Anyone who runs their own business sets themselves apart from the rest. Anyone who dares stick their neck out distinguishes themselves even more. That can be rather lucrative.
-
Tax technology
Driven by tax technology, we help you with your (most important) tax risks. Identify and manage your risks and become in control!
-
Transfer pricing
The increased attention for transfer pricing places greater demands on the internal organisation and on reporting.
-
Sustainable tax
In this rapidly changing world, it is increasingly important to consider environmental impact (in accordance with ESG), instead of limiting considerations to financial incentives. Multinational companies should review and potentially reconsider their tax strategy due to the constantly evolving social standards
-
Pillar Two
On 1 January 2024 the European Union will introduce a new tax law named “Pillar Two”. These new regulations will be applicable to groups with a turnover of more than EUR 750 million.
-
Cryptocurrency and digital assets
In the past decade, the utilization of blockchain and its adoption of a distributed ledger have proven their capacity to revolutionize the financial sector, inspiring numerous initiatives from businesses and entrepreneurs.
-
Expand into new markets
Do you seek for opportunities in the global business arena? Whether you are about to open a new office in a foreign country or considering an international acquisition, you need certainty of making the right choices for your company. Global expansion isn’t always as simple as it sounds. The good thing is that we’re here to help!
-
Expanding your business in the Netherlands
International expansion is an important step. The Netherlands can be your gateway to Europe for doing business abroad. But why you should choose the Netherlands?
-
Global contacts
Wherever you choose to do business, you want access to people with the best ideas and critical thinking that will enable you to grow your business at home and abroad.
-
Corporate Law
From the general terms and conditions to the legal strategy, these matters need to be watertight. This provides assurance, and therefore peace of mind and room for growth. We will be pro-active and pragmatic in thinking along with you. We always like to look ahead and go the extra mile.
-
Employment Law
Small company or large multinational: in any company your people are of the utmost importance for your business. Employment brings with it many issues in many areas and often has legal consequences. For big strategic, but also for more everyday questions about employment law, our lawyers are ready to help you out. Also for questions about international employment law. Do you have your own HR department? We’ll gladly assist them. We deliver bespoke services and are there when you need us.
-
Specialist Areas
Besides our focus on corporate and employment law, we also advise entrepreneurs on a range of (specialist) legal issues. A corporate acquisition, your company administration, complex question in the field of healthcare issues: you have come to the right place.
-
Sustainable legal
Sustainability is more than a buzzword - it is the core of our legal advice towards sustainable success. From drafting sustainable contracts, integrating sustainable HR policies and ESG due diligence within our M&A practice to advising on ESG and other (national and international) legislation: we prefer to be pragmatic and proactive in helping your business.
-
Maritime sector
How can you continue to be a global leader? The Netherlands depends on innovation. It is our high-quality knowledge which leads the maritime sector to be of world class.
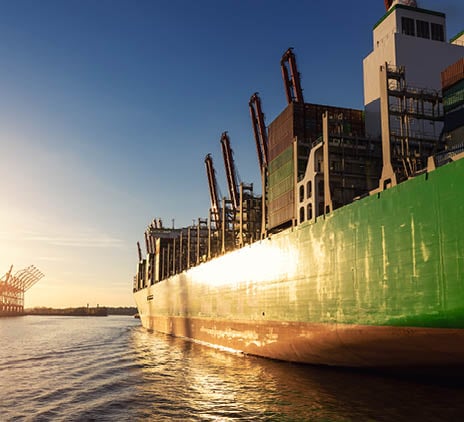
-
Growth in an international network
At Grant Thornton, you will benefit from the expertise and quality of colleagues around the world who will benefit your knowledge, advice and growth.
-
Varied customer portfolio
The customer package at Grant Thornton varies from (large) SME customers to (small) corporate customers. From local customers to customers from the international network of Grant Thornton International Ltd. All this diversity in customers can also be recognized in your customer package.
-
Culture
At Grant Thornton we combine a solid base with a flexible and results-driven mentality.
Data Strategy and Data Architecture
1. Data Governance
The key principles of data governance are ownership, responsibility, and accountability for data. This includes sharing information and consulting with relevant stakeholders on how the data can be used, processed, and stored. Setting up the appropriate data domains per product or business unit helps to manage information and maintain data integrity.
2. Data Quality
"Garbage in, garbage out." If an organization has the ambition to implement predictive analytics in the future, this is the first important step towards achieving that. When Machine Learning (ML) models are applied to data that is not of good quality, the chances of obtaining good results from these analyses are low. As this step is crucial, we develop appropriate controls to identify errors in the data and to make proposals to supplement or resolve them.
3. Data Lineage
Data lineage is the journey that data takes from the moment it is created. If all data flows are aligned and aligned with each other, any errors and changes in the data can be more easily detected and adjusted.
4. Data Architecture
Data architecture is a set of (policy) rules, models, and standards that determine how data is collected, stored, managed, and integrated in relation to database systems.
A good architecture enables us to truly rely on master data. Having a single source of truth is essential for analyses, business intelligence, repeatable automated processes, and machine learning/AI models.
5. Data quality solutions (data issue remediation)
Based on the points identified for improving data quality, a plan for implementation can be developed. One of the biggest challenges is determining what good data quality is for a particular application. This requires a deep understanding of the organization's data needs and requirements and the specific application.
In addition, obtaining good data quality can be challenging due to the diversity and complexity of data sources and types, the need for data integration and transformation, and the limited resources available for data management.
6. Unlocking complex data sources (data execution plans)
In recent years, the number of applications generating data has increased. Many organizations find unlocking data from these different sources to be complex. In addition to the technical complexity of unlocking data, it is important to create data execution plans that ensure that the data sources do not create timing issues.
Once all sources are unlocked and refreshed, the data can be linked together. The enterprise's data landscape determines the approach. If the data sources generate a lot of data and the enterprise's information needs are significant, there must be a central place where the data can be linked and transformed. This also applies when the enterprise wants to link several simple Excel files together and automate the analysis. When new data is obtained, it can be viewed in one place.
With your information needs as a guide, we prepare the data in such a way that we can use scripts to convert, combine, transform, or store the data in a central environment. This allows us, together with you, to build fast and thorough dashboards that answer your information needs.